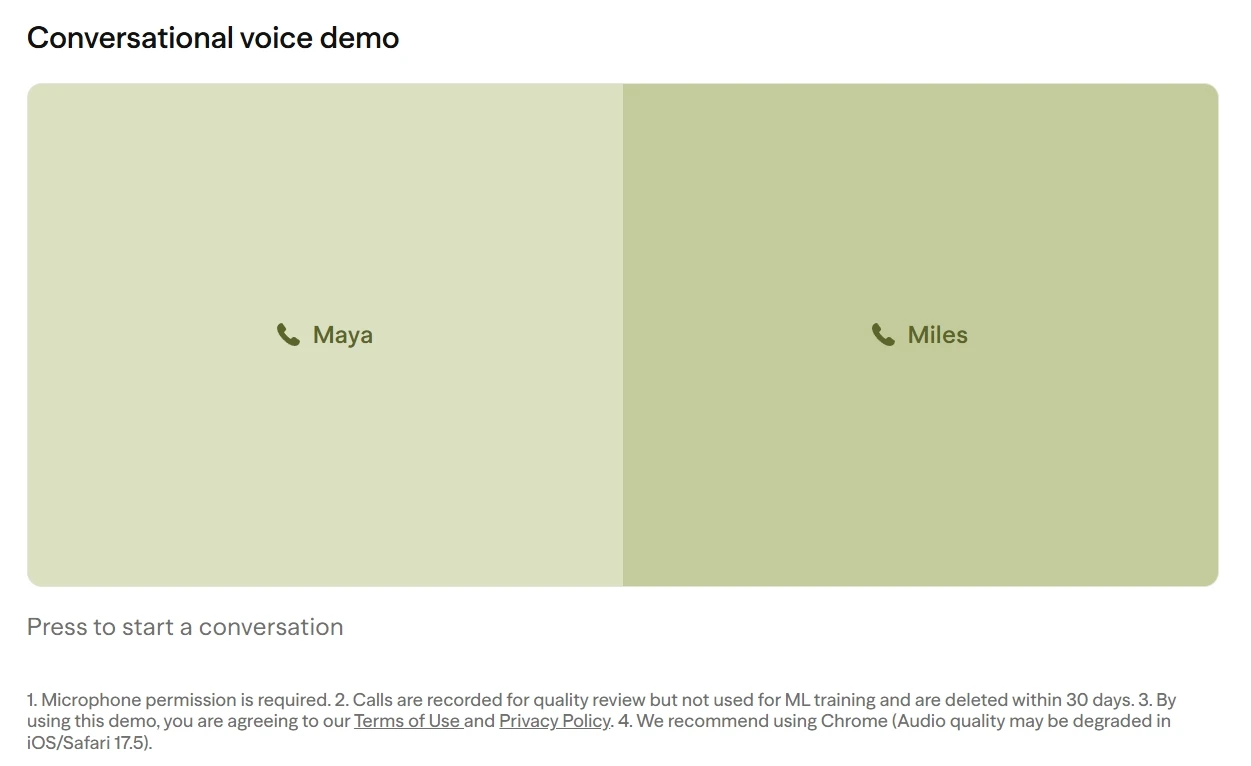
1. Core objectives
Sesame in through technological breakthroughs, so that voice assistants with natural, emotional interaction capabilities, across the "Valley of Horror" effect, to achieve a true "voice presence" (Voice Presence), so that the machine dialogue closer to the human The sense of reality and trust in communication.
2. Key technical challenges
- Emotion and context are missing: Existing voice assistants lack emotional expression, dialog pacing, and contextual adaptation, resulting in a stiff interaction.
- multimodal understandingThe traditional TTS model can hardly be adapted to the dynamic conversation scenarios as it needs to process multi-dimensional information such as text, speech, and emotion simultaneously.
- Real-time and efficiency: Traditional two-stage speech synthesis (semantic → acoustic) suffers from latency problems and cannot satisfy real-time interaction requirements.
3. Solution: Conversational Speech Model (CSM)
- End-to-end multimodal architecture::
- backbone network: Llama-based Transformer processes text and audio tokens to predict the underlying semantic tokens (layer 0).
- codec: Layered generation of residual acoustic tokens (layers 1 through N-1) with low-latency generation support.
- RVQ tokenization: Decompose speech into semantic tokens (high-level features) and acoustic tokens (detailed features), and optimize the generation efficiency by residual vector quantization (RVQ).
- Calculate the amortization strategy: Predicting acoustic tokens for only 1/16 audio frames during training reduces memory consumption while maintaining generation quality.
4. Experimentation and evaluation
- data setThe company's English speech data includes 1 million hours of English speech data, covering scenarios such as conversations and emotional expressions.
- model size::
- Tiny: 1B Backbone + 100M Decoder
- Small: 3B Backbone + 250M Decoder
- Medium: 8B Backbone + 300M Decoder
- Objective indicators::
- WER (Word Error Rate): Close to human levels (Small model 2.9%).
- Speaker similarity: 0.938 (close to the human benchmark of 0.940).
- new indicator::
- disambiguation of homonyms(e.g., "lead" pronunciation distinction): 871 TP3T accuracy for the Medium model.
- consistency of pronunciation(e.g., different pronunciation variants of "route"): Medium model 70%.
- Subjective evaluation (CMOS testing)::
- context-free: Human and CSM-Medium preference rates were close (47.11 TP3T vs 52.91 TP3T).
- context-sensitive: Human recordings significantly outperformed the model (66.71 TP3T vs. 33.31 TP3T), suggesting that contextual adaptation still needs to be improved.
5. Open source and future plans
- expand one's financial resources: Open source the model code and key components under the Apache 2.0 protocol to promote community collaboration.
- limitations::
- Reliance on English data with limited multilingual capabilities.
- Underutilized pre-trained language modeling knowledge.
- Inadequate modeling of conversational structures (e.g., turn-taking, pauses).
- future direction::
- Expanded support for 20+ languages and added multimodal training data.
- Exploring the fusion of pre-trained language models with speech models.
- Development of a full-duplex dialog model to implicitly learn dialog dynamics (e.g., pacing, pauses).
6. Summary
Sesame's CSM model has made a breakthrough in speech naturalness, but there is still room for improvement in contextual understanding and multilingual support. In the future, we need to promote voice assistants to move towards a more realistic and intelligent interaction experience through model scale extension, multimodal fusion and dialog structure modeling.
-
¥Download for freeDownload after commentDownload after login
- {{attr.name}}:
📢 Disclaimer | Tool Use Reminder
1️⃣ The content of this article is based on information known at the time of publication, AI technology and tools are frequently updated, please refer to the latest official instructions.
2️⃣ Recommended tools have been subject to basic screening, but not deep security validation, so please assess the suitability and risk yourself.
3️⃣ When using third-party AI tools, please pay attention to data privacy protection and avoid uploading sensitive information.
4️⃣ This website is not liable for direct/indirect damages due to misuse of the tool, technical failures or content deviations.
5️⃣ Some tools may involve a paid subscription, please make a rational decision, this site does not contain any investment advice.